Today morning at my parent's home, I asked Alexa to tell me the time of arrival of a flight. Alexa responded back with absolute precision. My parents were stunned! They wanted to know what made this device give a relevant answer.
The simple answer to their query is that services like Alexa are all powered by extensive knowledge graphs that allow the questions to be mapped to an organized set of connected and networked information that can often provide relevant answers. In some ways they work like our brain does, processing connected information, and pulling out the context specific network of nodes and relationships from a very large connected graph
Let’s move into the world of business and enterprise! Everyone will agree it’s so difficult to find answers to our queries and we spend enormous amount of time, energy and money in trying to get that relevant answer which drives our business. Can we replicate services like Alexa to our world of business so that we may get relevant answers with desired precision? Not just for simple problems but also for complex problems like understanding customer preferences in a very dynamic environment, fraud analysis, master data management, supply chain optimization, logistics analysis and optimization, investigating and solving complex crimes, new drug discovery process, identifying fake news, finding crime rings, and patterns.
The answer to above is yes and the technology that has made his possible is Enterprise Knowledge Graph.
Enterprise Knowledge Graph
To a layman a knowledge graph is a systematic way of putting data related to facts, people, business, location, events and many other attributes including transactional and preferences, to create interconnected visual results that are more accurate and relevant.
To an organisation a Knowledge Graph is a graphical representation of an organization’s knowledge domain and documents the representation in a way that is understood by both humans and machines.
In a more tech terminology, it is a collection of indexes which points to the organization’s knowledge assets, content, and data that leverages a data model to describe how these are related to the people, places, and things. It provides a structure and common interface for an organisation’s data and enables the creation of smart multilateral relations throughout the databases. Structured as an additional virtual data layer, the Knowledge Graph lies on top of the existing databases or data sets to link all data together at scale – be it structured or unstructured.
One of the best examples of knowledge graphs is the Google’s implementation of search. When you search for “Neo4J” in Google, the search engine not only provides the traditional search results, but since it understands that you are searching for the specific thing Neo4J, it provides an info-box, or knowledge panel, along with the traditional search results with specific information about the unique person as well as his or her relationships to other known objects in the graph such as License, java, as well as recommendations about similar products. The image below shows this example from Google.
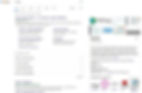
However, the Knowledge Graph of Google is quite limited in how users and software agents can interact with it. As it is in public domain the google knowledge graph limits itself to few areas of industry-specific knowledge, and it doesn’t cover the internal knowledge of companies. Its API returns only individual matching entities, rather than graphs of interconnected objects.
This is where the use cases of enterprise knowledge graphs come into play. The Knowledge graphs help organisations to create a knowledge web or knowledge graph representing the organisation its assets, connections and what not! The organisations using Enterprise Knowledge graphs can connect all data silos in a way that they become part of information highway, which can be used in a very agile way. The beauty of Enterprise Knowledge Graph is that it boosts existing IT infrastructure and helps an organisation create more value from it.
Many organisations in today’s fast changing world have already started using Knowledge graphs to gain competitive advantage and remain ahead in the game. Use of knowledge graphs has been in vogue in almost all industry verticals ranging from banking to pharma to retail to media and many more. The use cases may differ, but the road map is same: connecting data available in various data silos and making it valuable so that it an be used and re used in meaningful and an intelligent way.
Power of Knowledge Graph
Knowledge graphs are extremely powerful. When they are based on semantic standards, it is possible to relate knowledge to language in a structured way. In this way, language can provide a way into the graph. You can match words to the concepts represented by the graph.
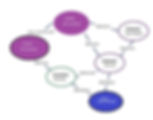
Knowledge graphs also allow you to create structures for the relationships in the graph. You can tell a graph that a company have employees and an employee can be a manager and two employees can be co-workers, and all of these are people. Providing such descriptive information allows new information to be inferred from the graph such as the fact that if two people have the same manager, they must be in the same group.
Because they are pictorial, knowledge graphs are more intuitive. People immediately understand graphs. When a knowledge graph is drawn, it makes sense to most people.
In addition, graphs are great at capturing scattered, unstructured, incomplete, and sketchy information, just like our brains. So, it is not that difficult to agree that having a knowledge graph of all the data in an organisation would be a good idea. But how would you get the data in? How would you find it when you need it? How do you manage it over time, allowing it to grow and change? To make knowledge graphs work in business, these questions must have good, complete answers. What we really need is a knowledge graph factory.
Limitations of today’s technologies in Business
Enterprises today have access to tools and technologies that collect and explore large data sets. But these are handicapped to give us an Alexa kind of experience, primarily because:
• Built in architecture is not suited to support graphs.
• Data models cannot support absorption of huge amount of data real time.
• Data extracts that power the discovery tools are too small.
Big data did attempt to address this space but the data integration that powers Alexa experience still did not happen.
The knowledge graph is the only currently implementable and sustainable way for enterprises to move to the higher level of integration needed to make data truly useful for a business along with great experience!
With a knowledge graph:
• The model can be made as large, as wide, and as deep as an enterprise want.
• Semantic standards are used to describe the structure of the information in the graph to support reasoning and inference.
• Both high-quality complete data, sparse and incomplete data can be captured and made useable.
The challenge is to create knowledge graphs that really work in the real-world creating value for the businesses.
Knowledge graph as Foundation of AI
One of the most powerful use of Knowledge graphs is powering AI applications with intelligence. In other words, knowledge graphs provide the context, the meaning behind various concepts in the data.
A knowledge graph helps the AI application understand that different entities in business have attributes that are specific to the entity. For example, difference between a human and a server. Moving a step further, knowledge graphs can power the applications to make inferences about the data, which may be the foundation for a recommendation engine, predictive analytics application or any other AI applications. These simple examples demonstrate the power behind teaching the AI machines in a way which is much similar to what we as humans think and do.
In summary, some of the key benefits of enterprise knowledge graphs are:
• Enterprise knowledge graphs help an enterprise to identify and collate all information in multiple and disparate data sources specific to an entity, person, line of business, products etc.
• The most in flavour and sought-after cognitive capabilities in an application can be powered by context from Enterprise knowledge graphs.
• Enterprise knowledge graphs help AI discover hidden facts and relationships through inferences in an enterprise’s integrated data that would otherwise be hidden and beyond reach.
A working knowledge graph
From the success Apple, Amazon, and Google who all have with graphs at scale, we know a knowledge graph is an asset for enterprises. All major search engines use them to make searches more intuitive. Social media giants use them to make user interaction more engaging. Start-ups have sprung to offer them as a service. Information providers, ranging from prominent newspapers to data vendors, are reshaping their data into Knowledge Graphs to open up new frontiers of data-driven applications. Several enterprises have creative pursuits underway, more often than not, in their nooks and crannies that target point applications. And a select few companies have built large Enterprise Knowledge Graphs that are use every day to solve hard business challenges at unmatched scales, efficiencies, and speeds.
From their experiences we know that knowledge graph does work and works well in the real world of business. Data is exploding. An enterprise knowledge graph makes all data usable, supporting the way we think and work.
Knowledge graph offers a clear path forward to a great experience in data management, transformation, and analysis that can keep up with the complexity and scale of the data present in a modern company.
References:
What is a Knowledge Graph? Transforming Data into knowledge. WP www. poolparty.biz
What Is An Enterprise Knowledge Graph and Why Do I Want One? Yanko Ivanov . November 1, 2018
2018: The Year of Enterprise Knowledge Graphs, Dan McCreary, Jan 1, 2018
Is The Enterprise Knowledge Graph Finally Going To Make All Data Usable? Dan Woods, January 1, 2018.
Knowledge Graphs: The Path to Enterprise AI,Michael Moore & Omar Azhar, Executive Director & Manager, EY | July 11, 2018